Challenge Sessions at ICIP 2024
This year’s ICIP Challenge sessions are sponsored by Technology Innovation Institute (TII).
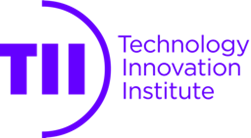
Challenge Session I: Monday 28 Oct 2024 | |
---|---|
13:15 - 13:30 | Overview of the Challenge Competetion Mohamed Deriche, Hassan Marzouki |
13:30 - 13:40 | Introduction to Challenge 1 Title: “Auto-WCEBleedGen Challenge Version V2” Nidhi Goel |
13:40 - 13:55 | Team 1 (Challenge 1) ColonNet: Auto-WCEBleedGen Challenge A.. Singh, S. Prakash, A. Das, Indian Institute of Information Technology, Ranchi |
13:55 - 14:10 | Team 2 (Challenge 1) Enhanced Detetcion and Segmentation of Bleeding Areas C. C. Hsu, C. M. Lee, Y. F. Lin, B. C. Qiu, National Cheng Kung University, Taiwan |
14:10 - 14:25 | Team 3 (Challenge 1) Failed Wizards, A Robust Pipeline for Classification of Bleeding Frames S. Alavala, Anil Kumar, A. Kancheti, S. Gorthi, IIT, Tirupati |
14:25 - 14:40 | Break |
14:40 - 15:00 | Introduction to Challenge 2 Title: “360-Degree Video Super-Resolution and Quality Enhancement Challenge” Wassim Hamidouche |
15:00 - 15:15 | Team 1 (Challenge 2) VACV, 360-Degree Video Super-Resolution and Quality Enhancement X. Sun, Y. Gao, J. Huang, and Y. Zhong are with Meituan Inc., China |
15:15 - 15:30 | Team 2 (Challenge 2) Dz360, 360-Degree Video Super-Resolution and Quality Enhancement Nassim Sehad1, Ahmed Saadallah2, Riku Jäntti1, Sidi-Mohammed Senouci2, and Mérouane Debbah3, 1Aalto University, 2University of Bourgogne, 3Khalifa University |
Challenge Session II: Monday 28 Oct 2024 | |
---|---|
16:00 - 16:20 | Introduction to Challenge 3 Title: “Grand Challenge on Video Complexity” Hadi Amirpour |
16:20 - 16:35 | Team 1 (Challenge 3) IVC1, Grand Challenge on Video Complexity J. Li, Yao Li, Z. Li, Li Li, Dong Liu, USTC, China |
16:35 - 16:50 | Team 2 (Challenge 3) IVC2, Grand Challenge on Video Complexity Yao Li, L. Junqi, Zhuoyuan Li, Li Li, L. Dong, USTC, China |
16:50 - 17:10 | Open Discussion and closing |
Located in Capital Suite – 19. Please find the details of the three challenges selected for ICIP 2024 below.
- Auto-WCEBleedGen Challenge Version V2
Prof Nidhi Goel, nidhi@igdtuw.ac.in
Overview
Gastrointestinal (GI) bleeding is a medical condition characterized by bleeding in the GI tract, which circumscribes oesophagus, stomach, small intestine, large intestine (colon), rectum, and anus. Since blood flows into the GI tract, a cascade of risks emerges, ranging from immediate dangers to potential long-term consequences. Excessive blood loss from GI bleeding may lead to a drop in blood pressure, reduced oxygen delivery to organs and tissues, and potentially life-threatening organ dysfunction.
According to World Health Organization (WHO), GI bleeding is responsible for approximately 300,000 deaths every year globally. These statistics serve as a catalyst for research, propelling innovative treatment modalities and diagnostic advancements aimed at mitigating the dangers posed by GI bleeding. In last decade, the availability of advanced diagnostic innovations like Wireless Capsule Endoscopy (WCE) has led to better understanding of the GI bleeding in GI tract. The disposable capsule-shaped device travels inside the GI tract via peristalsis and comprises of an optical dome, a battery, an illuminator, an imaging sensor, and a radio-frequency transmitter. During 8-12 hours of WCE procedure, a video of the GI tract trajectory is recorded on a device attached to the patient’s belt which produces about 57,000-1,00,000 frames; analysed posterior by experienced gastroenterologists.
Presently, an experienced gastroenterologist takes approximately 2−3 hours to inspect the captured video of one-patient through a frame-by-frame analysis which is not only time-consuming but also susceptible to human error. In view of the poor ratio of patient-to-doctor across globe, there arises a need for investigation and state-of-the-art development of robust, interpretable and generalized Artificial Intelligence (AI) models. This will aid in reducing the burden on gastroenterologists and save their valuable time by computer-aided classification between bleeding and non-bleeding frames and further detection and segmentation of bleeding region in that frame.
Auto-WCEBleedGen Challenge Version V1 was a huge success with +1200 participation across globe. It was organized virtually by MISAHUB (Medical Imaging and Signal Analysis Hub), in collaboration with the 8th International Conference on CVIP (Computer Vision and Image Processing 2023), IIT Jammu, India from August 15 – October 14, 2023. It focused on automatic detection and classification of bleeding and non-bleeding frames in WCE.
Following its success, we bring to you, Auto-WCEBleedGen Challenge Version V2 which focuses on automatic classification of bleeding and non-bleeding frames and further detection and segmentation of bleeding region in that frame. We have updated the annotations of the multiple bleeding sites present in the training dataset (WCEBleedGen). We have also updated the annotations and class labels of the testing dataset (Auto-WCEBleedGen Test) and provided un-marked images of dataset 1.
Organizing Team
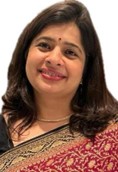
Dr. Nidhi Goel
Dept. of ECE
IGDTUW, Delhi
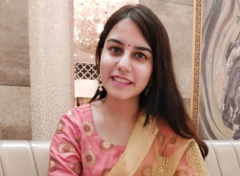
Palak Handa
Dept. of ECE
DTU, Delhi
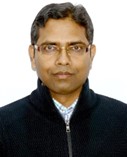
Dr. Deepak Gunjan
Dept. of Gastroenterology and HNU
AIIMS Delhi
- 360-Degree Video Super Resolution and Quality Enhancement Challenge
Prof Wassim Hamidouche, wassim.hamidouche@tii.ae
Overview
Omnidirectional visual content, commonly referred to as 360-degree images and videos, has garnered significant interest in both academia and industry, establishing itself as the primary media modality for VR/XR applications. 360-degree videos offer numerous features and advantages, allowing users to view scenes in all directions, providing an immersive quality of experience with up to 3 degrees of freedom (3DoF). When integrated on embedded devices with remote control, 360-degree videos offer additional degrees of freedom, enabling movement within the space (6DoF). However, 360-degree videos come with specific requirements, such as high-resolution content with up to 16K video resolution to ensure a high-quality representation of the scene. Moreover, limited bandwidth in wireless communication, especially under mobility conditions, imposes strict constraints on the available throughput to prevent packet loss and maintain low end-to-end latency. Adaptive resolution and efficient compression of 360-degree video content can address these challenges by adapting to the available throughput while maintaining high video quality at the decoder. Nevertheless, the downscaling and coding of the original content before transmission introduces visible distortions and loss of information details that cannot be recovered at the decoder side. In this context, machine learning techniques have demonstrated outstanding performance in alleviating coding artifacts and recovering lost details, particularly for 2D video. Compared to 2D video, 360-degree video presents a lower angular resolution issue, requiring augmentation of both the resolution and the quality of the video. This challenge presents an opportunity for the scientific research and industrial community to propose solutions for quality enhancement and super-resolution for 360-degree videos.
Organizing Team
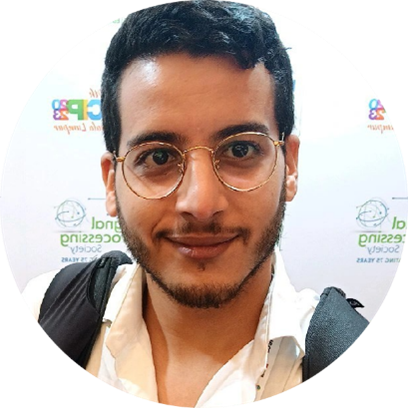
Ahmed Telili
Technology Innovation Institute
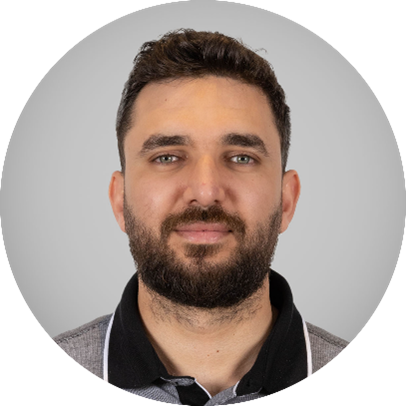
Dr Ibrahim FARHAT
Technology Innovation Institute
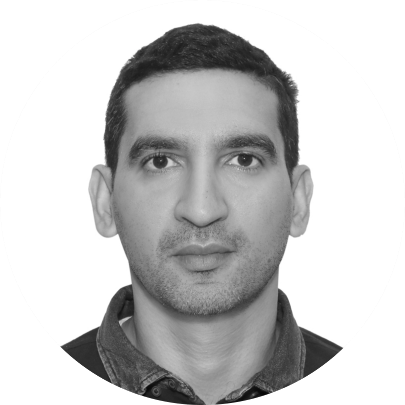
Dr. Wassim Hamidouche
Technology Innovation Institute
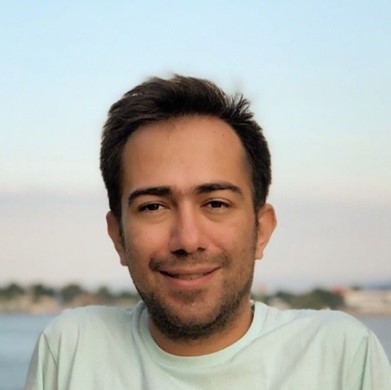
Dr Hadi Amirpour
Universität Klagenfurt
- Grand Challenge on Video Complexity
Prof. Hadi Amirpour, hadi.amirpour@aau.at
Overview
Video compression standards rely heavily on eliminating spatial and temporal redundancy within and across video frames. Intra-frame encoding targets redundancy within blocks of a single video frame, whereas inter-frame coding focuses on removing redundancy between the current frame and its reference frames. The level of spatial and temporal redundancy, or complexity, is a crucial factor in video compression. Generally, videos with higher complexity require a greater bitrate to maintain a specific quality level. Understanding the complexity of a video beforehand can significantly enhance the optimization of video coding and streaming workflows. While Spatial Information (SI) and Temporal Information (TI) are traditionally used to represent video complexity, they often exhibit low correlation with actual video coding performance. In this challenge, the goal is to find innovative methods that can quickly and accurately predict the spatial and temporal complexity of a video, with a high correlation to actual performance. These methods should be efficient enough to be applicable in live video streaming scenarios, ensuring real-time adaptability and optimization.
Organizing Team
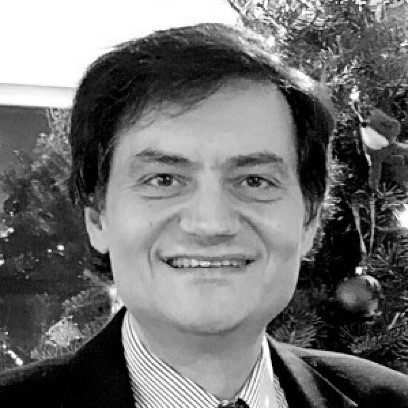
Dr Loannis Katsavounidis
Facebook
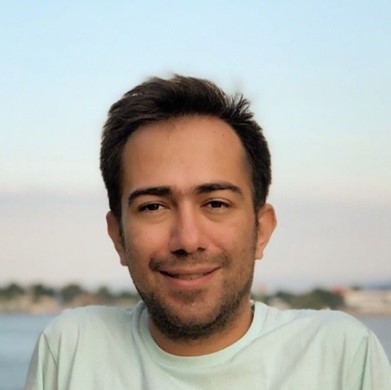
Dr Hadi Amirpour
Universität Klagenfurt
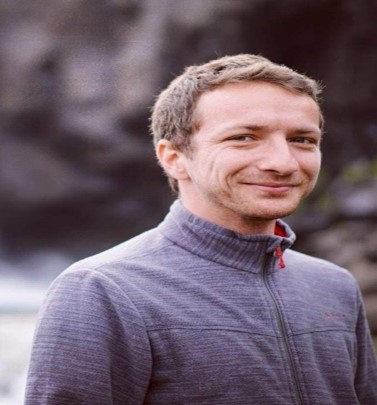
Dr Ali Ak
Nantes University
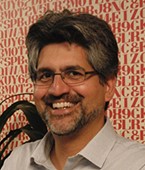
Dr Anil Kokaram
Trinity College Dublin.
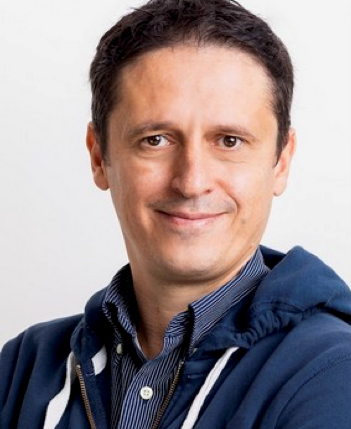
Dr Christian Timmerer
Universität Klagenfurt
For any general questions regarding the challenges, kindly contact the Challenge Co-Chairs (challenges@2024.ieeeicip.org).